AI and data literacy
AI literacy
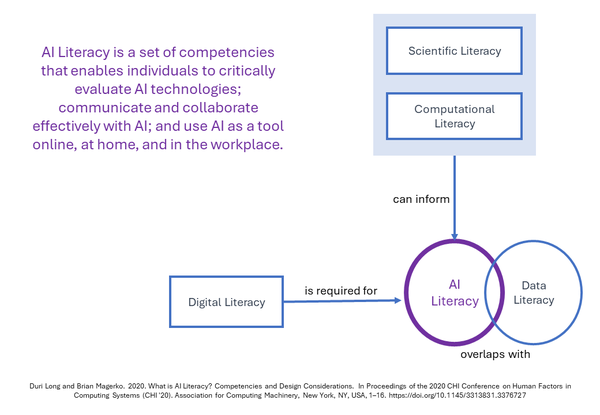
AI literacy (alongside data/digital literacy)
Scope:
- Applicable to the entire workforce, from entry-level to executive roles
- Requires organisation-wide education, training and awareness initiatives
Impact:
- Enables effective communication and collaboration around AI initiatives
- Helps employees understand the implications of AI on their roles and the organisation
- Facilitates informed decision-making and responsible use of AI technologies
Applying SFIA to AI and data literacy
- The SFIA levels of responsibility and the generic attribute for knowledge provide strong guidance on what organisations should look for in data, digital or AI literacy measurement scales
- It's important to note that the measurement scales used for literacy and fluency are different from SFIA's levels of responsibility, even if they sometimes appear similar (e.g., using a numeric scale).
- Literacy and fluency measurement scales can be used alongside SFIA, but the distinction between the two should be made clear. E.g. it can be helpful not to use a numeric scale for literacy / fluency to avoid confusion between SFIA's 7 levels.
AI/Data literacy and SFIA Levels are "independent but complementary"
It can be more useful and realistic to consider the relationship between AI/Data literacy and SFIA Levels as "independent but complementary" or orthogonal. This means:
- No direct correlation: Higher SFIA levels do not automatically require higher AI literacy levels.
- Role-specific requirements: The appropriate AI literacy level depends on the specific role and its interaction with AI, not just seniority.
- Strategic vs. Technical: Senior roles (SFIA 6-7) may need "Working" or "Practitioner" literacy rather than "Expert" if their focus is strategic rather than technical.
- Domain-specific application: Within the same SFIA level, different specialisations will require different AI literacy levels.
Illustration of relationship between AI/Data literacy and SFIA levels
Here is a hypothetical (but realistic) scale for AI/Data literacy
Level |
Key focus |
Development approach |
Awareness |
Basic understanding of what AI is and its applications |
Introductory workshops, general awareness sessions |
Working |
Regular effective use and configuration of AI tools, basic critical evaluation |
Tool-specific training, guided implementation activities |
Practitioner |
Selection and implementation of appropriate AI solutions, thorough evaluation |
Advanced workshops, practical implementation projects |
Expert |
Create, customise, or evaluate AI solutions at a technical level. Innovation and technical leadership. |
Specialised technical training, research and development activities |
Mapping different (illustrative) roles against SFIA Levels and AI/Data literacy could look like this
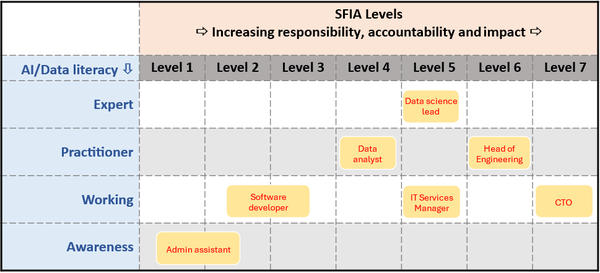
- This graphic shows that senior roles may not need expert literacy - SFIA Level 7 role might only need working-level because their role focuses on strategic decisions rather than hands-on AI implementation.
- Focusing on role function rather than hierarchy - The framework assesses literacy requirements based on how a role interacts with AI (creating, using, or making decisions about AI) rather than its position in the organisational hierarchy.
This raises a crucial question with this type of AI/data literacy scale .
- How can a SFIA level 7 seem to require lower levels of AI literacy than other roles?
This can be explained by considering an additional aspect of AI/data literacy - application of strategic expertise
- The level 7 role requires strategic transformation capabilities
- The level 7 doesn't need to personally implement technical solutions
- Technical depth and strategic breadth are different but equally valuable knowledge areas
-
- Implementation Track: Focuses on technical use and development of AI (Working → Practitioner → Expert)
- Strategic Track: Focuses on business application and organizational integration (Informed → Direction-Setting → Transformational)
The Dual-Track framework naturally explains why a CTO at SFIA Level 7 would be at the "Transformational" level on the Strategic track (high) while only needing "Working" level on the Implementation track (not high).
Catering for strategic expertise
A dual track approach will help to communicate and clarify that strategic application isn't an extension of technical expertise but rather a parallel developmental path.
Targeted Development for Strategic Roles
- With this framework, we can create more targeted development for SFIA 6-7 roles by focusing on advancing their Strategic track capabilities rather than trying to deepen their technical implementation skills.
- For example, a CTO development program would focus on:
- Industry disruption analysis
- Business model transformation through AI
- Strategic investment frameworks
- Technology horizon scanning
- Ecosystem development
- Rather than:
- Advanced model development techniques
- Technical implementation details
- Coding practices
Benefits of This Approach
- Conceptual Clarity: Clearly separates strategic application from technical implementation
- Appropriate Development: Focuses learning and professional development on the right track for each role
- Equal Value: Recognizes that both tracks represent valuable but different skills
- Flexible Progression: Supports different career paths and specialisation
This Dual-Track approach recognises strategic application as a distinct developmental path rather than an extension of technical expertise. It maintains the integrity of our existing scale while providing a clearer framework for developing the strategic capabilities needed for AI/data transformations at higher SFIA levels.
SFIA skills for learning and development professionals
- SFIA provides several skills for learning and development professionals involved in building AI and data literacy
SFIA skill - name |
Code |
SFIA skill - concise description |
Illustrative applications |
AI and data ethics | AIDE | Implementing and promoting ethical practices in the design, development, deployment, and use of AI and data technologies. |
Ensuring AI systems used for patient diagnosis comply with ethical standards to protect patient privacy and ensure unbiased outcomes. Developing an AI ethics training program for employees involved in AI projects to ensure responsible AI development and deployment. |
Learning design and development | TMCR | Designing and developing resources to transfer knowledge, develop skills and change behaviours. | Creating an interactive e-learning course on AI fundamentals for employees across different departments to build a foundation of AI literacy. |
Content design and authoring | INCA | Planning, designing and creating textual information, supported where necessary by graphical content. |
Writing a series of articles or blog posts explaining key AI concepts and their applications in various business contexts to raise awareness and understanding. |
Learning delivery | ETDL | Transferring knowledge, developing skills and changing behaviours using a range of techniques, resources and media. |
Workshops: Conducting interactive workshops where employees learn about AI through hands-on activities and collaborative projects. Webinars: Hosting online seminars to teach remote employees about AI developments and their implications for the business. |
Competency assessment | LEDA | Assessing knowledge, skills, competency and behaviours by any means, whether formal or informal, against frameworks such as SFIA. | Designing assessments to measure employees' understanding of AI concepts and their ability to apply AI tools in their daily tasks. |
Overlap between AI literacy and data literacy
Understanding of data
- Common ground: Both AI literacy and data literacy require individuals to understand how data is collected, processed, and analysed.
- Data literacy focus: Reading, understanding, and communicating data within decision-making processes.
- AI literacy focus: Extends data literacy capabilities to include comprehension of how data informs AI models, influencing automated decisions and predictive analytics.
Critical evaluation
- Data evaluation: Essential for critically evaluating sources of data and the outputs from data analysis.
- AI evaluation: Involves evaluating the outputs of AI models, understanding their limitations, biases, and the ethical considerations of their application.
Utilisation of tools
- Data literacy tools: Involves using tools and software for basic data analysis.
- AI literacy tools: Encompasses the use of AI platforms and tools that automate or provide more advanced analyses.
Decision-making and problem-solving
- Enhanced decision-making: Both literacies contribute to more informed and effective decision-making processes.
- AI augmentation: AI literacy allows for leveraging AI technologies to automate and augment decision-making, especially in complex scenarios involving predictive analytics.
Integration and synergy
- Integrated training: Promotes an integrated approach to employee training and workflow design, empowering employees to leverage data effectively.
- Workforce proficiency: Supports the creation of a workforce proficient in navigating the complexities of modern data landscapes and AI technologies, enhancing organisational productivity and innovation.
Digital literacy as a foundation for AI literacy
Summary: Digital literacy is not just a complementary skill but a prerequisite for AI literacy. It equips individuals with the fundamental understanding and skills needed to operate in a digital environment, essential for effectively using AI technologies.
Basic technology skills
- Essential skills: Digital literacy provides the skills needed to use computers, smartphones, and other digital devices necessary for engaging with more advanced technologies like AI.
- Navigational skills: Includes navigating operating systems, using common software applications, and understanding the internet and cloud services, integral to operating AI-powered tools.
Understanding of digital content
- Content management: Digital literacy involves understanding how digital content is created, managed, and manipulated, crucial for comprehending how data is used and manipulated by AI systems.
- AI interaction: Being literate in digital content allows individuals to discern how AI models are trained with data and how these models generate outputs, making it easier to interact with AI applications effectively.
Critical thinking and online communication
- Evaluation and communication: Digital literacy enhances critical thinking and effective online communication, essential competencies for engaging with AI.
- Collaborative environments: Users must critically evaluate data-driven outputs, understand their sources, and communicate findings or discrepancies effectively, especially in collaborative environments that use AI.
Safety and security online
- Digital security: Understanding digital security is vital for AI literacy, particularly because AI systems frequently process sensitive data.
- Privacy and cybersecurity: Knowledge of data privacy, cybersecurity practices, and data integrity helps users navigate the security implications of AI technologies, ensuring secure interactions and compliance with legal and ethical standards.
Seamless transition to AI tools
- Adaptability: Digital literacy facilitates a smoother transition to using AI tools by providing users with the skills to adapt quickly to new technologies.
- Effective usage: Digitally literate individuals are more likely to effectively use and troubleshoot AI applications, integrate them into daily tasks, and maximize their potential benefits in various contexts.